Model Intercomparison and Accuracy Assessment
OpenET has conducted one of the largest intercomparison and accuracy assessments of field-scale satellite-driven ET models to date.Satellite-derived OpenET data were compared against ET measurements collected by 151 flux tower stations and four precision weighing lysimeters located throughout the continental U.S. The key results for croplands are summarized below, and information for other land cover types will be provided in the OpenET Intercomparison and Accuracy Assessment Report, along with the technical details of the methods used in the assessment. Descriptions of the methods used to calculate the satellite-derived ET values are available in the Methodologies page.
For croplands, the ensemble performed as well as or better than any individual model across most accuracy metrics, though nearly all models demonstrated high accuracy measures for croplands. Accuracy metrics for the slope of the best fit line through the origin (slope), mean bias error (MBE), mean absolute error (MAE), root mean squared error (RMSE), and r-squared (r2) value are summarized in the table below for the water year (October-September), growing season (determined dynamically, as described in the full report), and monthly and daily timesteps. Descriptions of each of these accuracy assessment metrics and how to interpret them are provided below.
Accuracy Summary for Croplands for the OpenET Ensemble ET Value
Time Period | Slope | Mean Bias Error) | Mean Absolute Error | Root Mean Squared Error | r-squared | Mean flux tower ET |
Water Year: 16 sites with 72 total water years |
0.91 | -73.91 mm (-7.5%) | 112.12 mm (11.3%) |
121.87 mm (12.3%) |
0.84 | 991 mm |
Growing Season: 39 sites with 177 growing seasons | 0.96 | -11.9mm (-2.0%) | 78.14 mm (12.9%) |
93.79 mm (15.5%) |
0.87 | 605 mm |
Monthly: 46 sites with 1,791 total months |
0.92 (n = 53) |
-5.27 mm (-5.8%) |
15.84 mm (17.3%) |
20.44 mm (22.4%) |
0.90 (n = 53) |
91 mm |
Daily: 55 sites with 5,508 total Landsat overpass days |
0.86 (n = 60) |
-0.35 mm (-10.0%) |
0.83 mm (23.6%) |
1.09 mm (31.1%) |
0.81 (n = 60) |
3.5 mm |
At annual timescales, 16 sites had data for at least one full water year, which required at least 12 consecutive months of data and a minimum of one cloud-free Landsat satellite observation per month. The data records for these 16 sites included 72 total water years. The OpenET ensemble value had a slope of 0.91, and MAE and RMSE values of <10%, with an r2 value of 0.84, demonstrating excellent overall agreement with the flux tower data. The MBE was -7.9%, which is still excellent, but indicates a small negative bias in the ensemble ET value relative to the ground-based ET, and this bias should be accounted for in evaluating annual ET totals.
For the growing season, 39 sites had at least one complete growing season with a total of 177 complete growing seasons across these sites. The OpenET ensemble value had a slope of 0.96, MBE of -2.0%, MAE of 12.9%, RMSE of 15.5%, and r2 of 0.87. These results demonstrate very strong agreement between the ensemble value and the ground-based ET datasets during the growing season. The slope of 0.96 and MBE of -2.0% indicates that on average, the ensemble ET value has almost no bias during the growing season, and when combined with the low MAE and MBE and high r2, demonstrates good accuracy across a wide range of crops and meteorological conditions.
At a monthly timestep, 44 sites (53 sites for slope and r2) had at least three full complete months of data with a minimum of one cloud-free Landsat overpass per month, and a total of 1,638 months in the dataset (1652 months for slope and r2). The OpenET ensemble value had a slope of 0.92, MBE of -5.8%, MAE of 17.3%, RMSE of 22.4%, and r2 of 0.90. The slope near 1.0, minimal bias error, and very high r2 indicate excellent overall accuracy for the ensemble ET value at monthly timescales with near-zero bias. The MAE of 17.3% and RMSE of 22.4% are still very good, but slightly above the OpenET target of <15% at a monthly timestep. The low bias error suggests that the higher MAE and RMSE are likely due to random error in both the satellite and ground-based datasets, as well as periodic error in the satellite data when monthly values are calculated from only one or two cloud-free satellite observations. The OpenET team will be working on integration of Sentinel-2, and future NASA and European Space Agency missions to increase the observation frequency, and further reduce the MAE and RMSE values at monthly timesteps.
At a daily timestep, 52 sites (60 sites for slope and r2) had at least 6 daily observations concurrent with satellite observations, with a total of 5,225 total paired daily estimates in the dataset (5,255 days for slope and r2). The OpenET ensemble value had a slope of 0.86, MBE of -10.0%, MAE of 23.6%, RMSE of 31.1%, and r2 of 0.81. These accuracy metrics are all very good, though as expected, at a daily timestep the influence of random error increases. The effects of outliers due to cloud contaminated pixels or model errors also have a stronger influence, especially during the winter months when ET rates are low. Overall, the slope, MBE, and r2 values are considered to be very good to excellent, and indicate good overall accuracy. The MAE and RMSE values were slightly above the OpenET target of 20%, and it will be important that this uncertainty is accounted for in irrigation management applications. The work described above to improve the monthly data products is expected to also improve the ensemble ET accuracy at daily timesteps.
For water resource management and water accounting applications, the low bias error at all timesteps indicates that over large areas, the random error that affects the monthly and daily data should partially cancel (Allen et al., 2007), and the OpenET ensemble value should be highly accurate. The bar graph below shows the average growing season ET across all flux tower sites (both closed and unclosed) along with the average ET for the ensemble value and each of the individual models. While all models agree very well and are within +/- 15% of the average flux tower ET, the OpenET ensemble value is within 2% (10 mm) of the average growing season ET measured by the flux towers, following correction for energy balance closure.
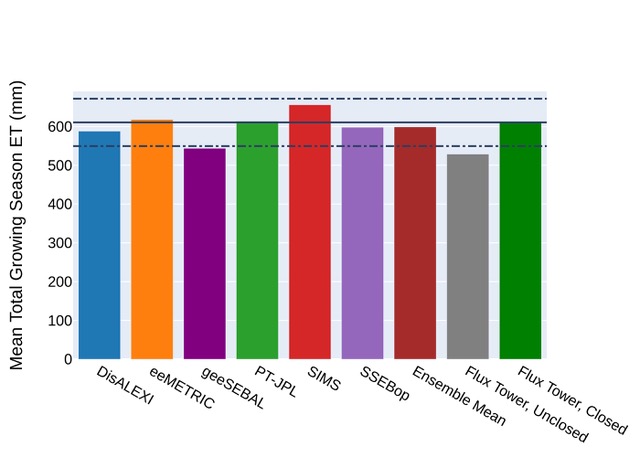
Average Total Growing Season ET (n=39 sites and 177 total growing seasons). The solid line indicates the mean closed station ET for croplands and the dashed lines represent +/- 10% of the mean.
In considering these results, it is important to note that most cropland sites were located in expansive regions with well-watered crops. In these regions, (including most of California’s Central Valley and Delta, and most agricultural regions in the Midwest), the ensemble value appears to provide the most reliable and stable estimate of ET. However, when looking at the limited number of cropland in-situ flux stations located in very arid environments, there is evidence that some models have a systematic low bias for smaller agricultural areas in very arid regions. In these areas, the MAD outlier filtering approach does not filter outliers as desired due to the large range in ET values from the ensemble relative to the ensemble median, resulting in a low bias in the ensemble ET value. Over the coming months, the team will continue to conduct additional research in these more challenging settings. As the ensemble calculation evolves based on this research, additional comparisons will be conducted and this page will be updated, along with our Best Practices Manual.
For more details and results for natural land cover types (evergreen forests, mixed forests, grasslands, shrublands, and wetland/riparian), see the OpenET Intercomparison and Accuracy Assessment Report. Accuracy metrics were more variable for some natural land cover types than for croplands, but demonstrated good overall accuracy. A positive bias in the ensemble ET was identified in evergreen forests, mixed forests, and wetlands, and monthly MAE and RMSE values were higher than the OpenET target accuracies, highlighting areas for future research. Quantifying the accuracy of both the individual models and the ensemble ET value is an important first step, and will allow water managers and agricultural producers to account for uncertainty in ET estimates when integrating data from OpenET into water management applications. An important part of the mission for OpenET.org is to continue advancing the underlying science, and the OpenET team will continue to improve the ensemble value and individual model accuracies.
Explanation of Accuracy Metrics
OpenET selected five key metrics to characterize the accuracy of OpenET data:
- Slope: The slope of the best-fit line forced through the origin provides a measure of overall agreement between the satellite data and ground-based ET data. A slope of less than 1.0 indicates that the satellite ET data are, on average, less than the ground-based ET data. A slope greater than 1.0 indicates that, on average, the satellite ET data are greater than the ground-based ET data. A slope of 1.0 indicates no overall bias, and values between 0.9 and 1.1 are generally considered excellent for remotely sensed ET.
- Mean Bias Error (MBE): As the name suggests, MBE provides a measure of overall bias and is closely related to the slope. MBE quantifies the bias in the satellite ET data relative to the ground-based ET data, and characterizes the expected overall error when the remotely sensed ET data are aggregated over large areas. A negative bias indicates that the satellite ET data are, on average, less than the ground-based ET data. A positive bias indicates that, on average, the satellite ET data are greater than the ground-based ET data. A value of zero would represent no bias, and values in the range of +/- 10% are considered excellent for remotely sensed ET.
- Mean Absolute Error (MAE): MAE provides a measure of the expected error for a given location and time period, and quantifies the average absolute difference between the satellite and ground-based ET data. Since MAE provides the absolute error, the value is often considered to characterize typical expected error both above and below the reference data. A value of zero represents perfect agreement and values in the range of 0-15% (interpreted as up to +/-15%) are considered excellent for remotely sensed ET data over agricultural lands. Accuracy requirements for remotely sensed ET vary by application, however, values in the range of 0-25% are considered to be very good and acceptable for many applications. MAE includes random error in both the satellite-based ET data and the ground-based ET data that are used as a reference. When MBE is much lower than MAE, it may indicate that random error in the satellite data is the primary contributor to error, or that error in the ground-based reference ET is also contributing to the MAE. When MAE and MBE are similar in magnitude, it usually indicates that error is due to a persistent bias in the satellite-based ET data.
- Root Mean Squared Error (RMSE): RMSE is another widely used measure of the expected error for a given time and location. It is interpreted in the same way as MAE, but provides greater weight to outliers or large ‘misses’ in the satellite-based ET data. RMSE may be used as a measure of expected error when the intended use of the data is sensitive to large errors in the satellite data for any given location or time period. A value of zero represents perfect agreement, and values in the range of 0-25% RMSE are generally considered to be very good for satellite-based ET data.
- r-squared: The r-squared value provides a measure of the proportion of the variance in the ground-based ET data that is explained or reproduced by the satellite-based ET data. It can be interpreted as a measure of the ability of the satellite-based ET to accurately estimate the relative magnitude of ET in the ground-based ET data. A value of 1.0 would mean that the satellite-based ET data explains 100% of the variance in the ground-based ET data. Values above 0.6 are considered to be very good, and values above 0.85 for remotely sensed ET data are considered to be excellent. It is important to note that a model can have an r-squared value near 1.0, and still have a consistent high or low bias.